Predicting tsunamis accurately is crucial for disaster preparedness, yet traditional methods rely on detailed bathymetric maps, which are often unavailable.
A research team led by Assistant Professor Justin Yen-Ting Ko tackled this challenge by developing a novel approach that eliminates the need for precise ocean floor data. Using ambient noise interferometry, they analyzed 10 years of background ocean noise recorded by the Deep-ocean Assessment and Reporting of Tsunamis (DART) system in the Pacific Ocean. From this data, they derived empirical Green’s functions of infragravity waves (IGWs), which closely mimic the behavior of tsunami waves in shallow water.
To validate their method, the team applied it to a real tsunami event in Alaska. Their predictions matched observed pressure data more accurately than traditional simulations using the Cornell Multi-Grid Coupled Tsunami Model. By removing the dependence on precise bathymetric information, this innovative technique offers a practical solution for tsunami forecasting in regions where detailed seafloor data is unavailable.
This breakthrough could enhance disaster preparedness, improve tsunami prediction accuracy, and ultimately help save lives.
The research was published in Geophysical Research Letters:
Ho, K.-C., Ko, J. Y.-T.*, Huang, H.-H., & Lee, S.-J. (2025). A novel approach to tsunami prediction using ambient noise-derived Green’s functions. Geophysical Research Letters, 52, e2024GL113971. https://doi.org/10.1029/2024GL113971
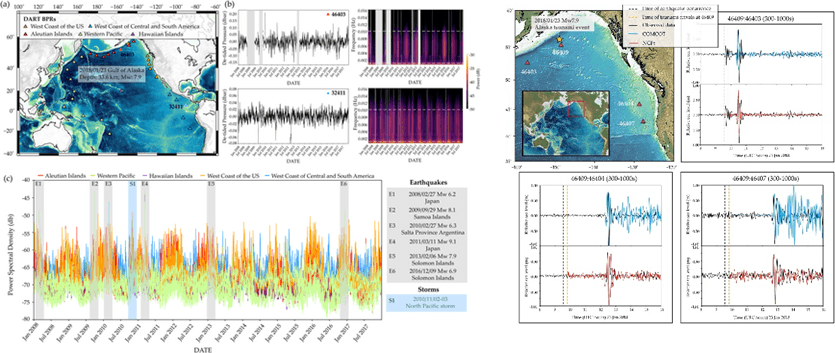
Figure 1. (Left) Global distribution of pressure gauge data and decadal variations in power spectral density (PSD) over the past ten years. (Right) Waveform fitting for a real tsunami event: Black: Observed data; Blue: COMCOT simulated waveform; Red: Waveform calculated using our proposed method.